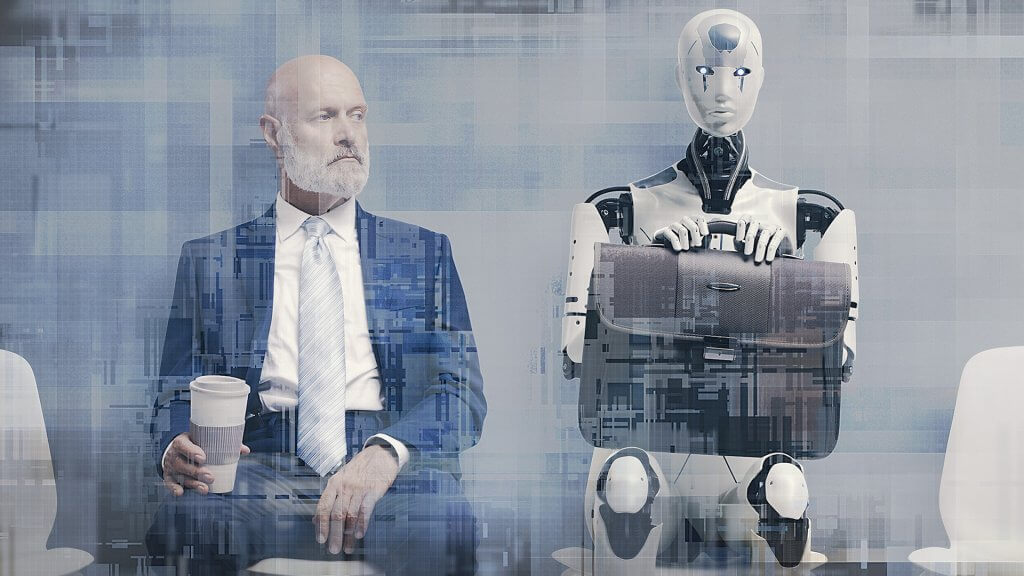
Artificial intelligence (AI) is evolving at a breakneck pace, transforming businesses and society. As we look towards 2024, it’s crucial to navigate the hype and set realistic expectations. In this article, we’ll explore the key AI trends shaping the future.
The Reality Check: Moving Beyond the Hype
AI tools like ChatGPT and DALL-E have captured the public’s imagination. However, it’s essential to understand their capabilities and limitations. The trend of embedded AI focuses on integrating AI into existing software and workflows.
By setting realistic expectations, we can harness AI as a tool for augmenting human capabilities. Imagine AI as a helpful assistant, like Siri or Alexa, rather than a replacement for human intelligence. Just as these virtual assistants enhance our daily lives, AI can boost productivity and efficiency in various industries.
For example, let’s say you’re a busy executive who needs to quickly summarize a lengthy report. Instead of spending hours reading through the document, you could use an AI-powered tool to generate a concise summary in minutes. This would allow you to grasp the key points and make informed decisions faster.
Similarly, imagine you’re a content creator looking to generate engaging social media posts. An AI writing assistant could help you brainstorm ideas, suggest catchy headlines, and even optimize your content for different platforms. By leveraging AI as a creative partner, you can save time and focus on crafting compelling stories that resonate with your audience.
However, it’s crucial to remember that AI is not a magic solution. It has limitations and can sometimes produce biased or inaccurate results. That’s why it’s essential to approach AI with a critical eye and use it as a supplement to, rather than a replacement for, human judgment.
As we navigate the hype surrounding AI, let’s keep our expectations grounded in reality. By understanding the strengths and weaknesses of AI tools, we can harness their potential to enhance our work and lives while staying mindful of their limitations.
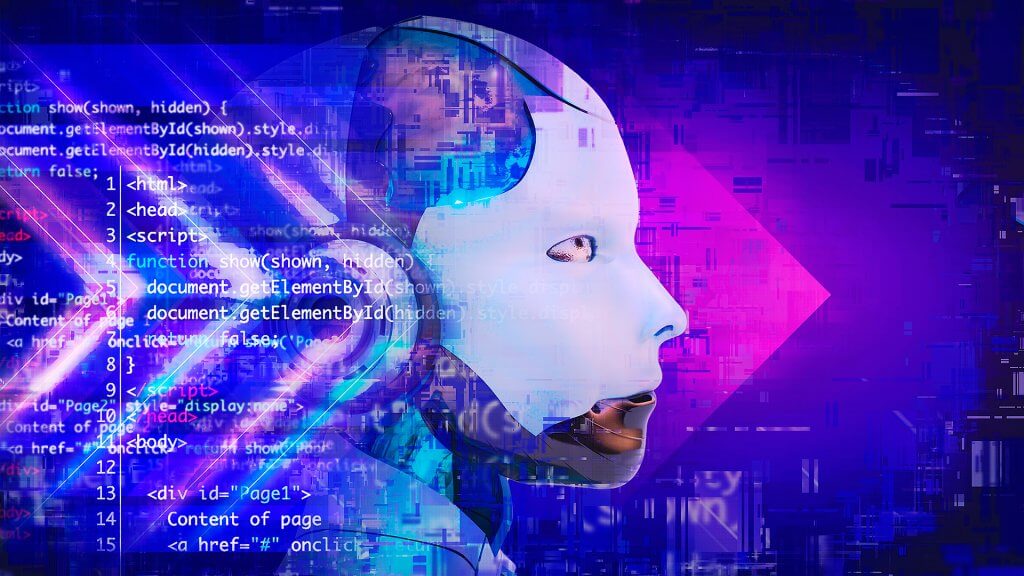
The Rise of Multimodal AI
Multimodal AI refers to AI models that can process and generate multiple data types. This includes text, images, audio, and video. The potential for more natural and intuitive human-machine interactions is vast.
Picture a virtual assistant that can not only understand your voice commands but also analyze images you share. It could help you identify objects, translate text, or even provide fashion advice based on your style preferences. However, developing multimodal AI systems comes with challenges, such as requiring large amounts of diverse training data.
Imagine you’re a language learner trying to improve your pronunciation. With multimodal AI, you could speak into your device’s microphone and receive real-time feedback on your accent, intonation, and fluency. The AI could analyze your speech patterns, compare them to native speakers, and provide personalized suggestions for improvement.
But the applications of multimodal AI go beyond personal use. In healthcare, for example, multimodal AI could revolutionize diagnosis and treatment. An AI system could analyze a patient’s medical images, such as X-rays or MRIs, while simultaneously considering their electronic health records, genetic data, and even lifestyle factors. By combining these diverse data points, the AI could provide a more comprehensive and accurate assessment of the patient’s condition.
However, building multimodal AI systems is no easy feat. It requires vast amounts of high-quality, diverse data to train the models effectively. This data must be carefully curated and labeled to ensure the AI learns the right associations and patterns.
Moreover, there are ethical considerations to keep in mind. As multimodal AI becomes more advanced, it can potentially be used for malicious purposes, such as creating deepfakes or manipulating public opinion. It’s crucial that we develop these technologies responsibly and put safeguards in place to prevent misuse.
Despite the challenges, the rise of multimodal AI represents an exciting frontier in human-machine interaction. By combining different data modalities, we can create AI systems that are more intuitive, context-aware, and responsive to our needs. As we continue to push the boundaries of what’s possible with multimodal AI, let’s do so with a commitment to ethics, transparency, and the greater good.
The Quest for Smaller and More Efficient Models
Large AI models, like GPT-3, have made headlines for their impressive capabilities. However, they come with significant environmental and computational costs. The trend towards developing smaller, more parameter-efficient models aims to address these concerns.
Imagine if your smartphone could run powerful AI applications without draining the battery or requiring an internet connection. Smaller models enable faster training, reduced costs, and improved accessibility. This means that even small businesses and individuals can leverage AI without breaking the bank.
Think about the last time you used a translation app on your phone. With smaller, more efficient AI models, you could have real-time translations without any lag or delay. The app could work seamlessly, even in areas with poor internet connectivity, making it easier to communicate with people from different language backgrounds.
But the benefits of smaller models go beyond convenience. They also have important implications for sustainability and inclusivity. Training large AI models consumes vast amounts of energy, contributing to carbon emissions and climate change. By developing more efficient models, we can reduce the environmental impact of AI and make it more sustainable in the long run.
Moreover, smaller models democratize access to AI. They lower the barriers to entry, allowing more people and organizations to benefit from this transformative technology. Imagine a small farm owner in a developing country who wants to use AI to monitor crop health and optimize yield. With affordable, efficient AI models, they could access these tools without needing expensive hardware or technical expertise.
However, creating smaller models is not without its challenges. It requires advanced techniques like knowledge distillation, pruning, and quantization to compress the models while maintaining their performance. Researchers and developers need to find the right balance between model size and accuracy.
Despite the challenges, the quest for smaller and more efficient AI models is a crucial step towards making AI more accessible, sustainable, and inclusive. By pushing the boundaries of what’s possible with limited resources, we can unlock the potential of AI for everyone, not just the privileged few.
So, the next time you use an AI-powered app on your phone, remember the incredible innovation that goes into making it small, fast, and efficient. And as we continue to develop smaller models, let’s keep in mind the bigger picture: a world where AI benefits all of humanity, not just a select few.
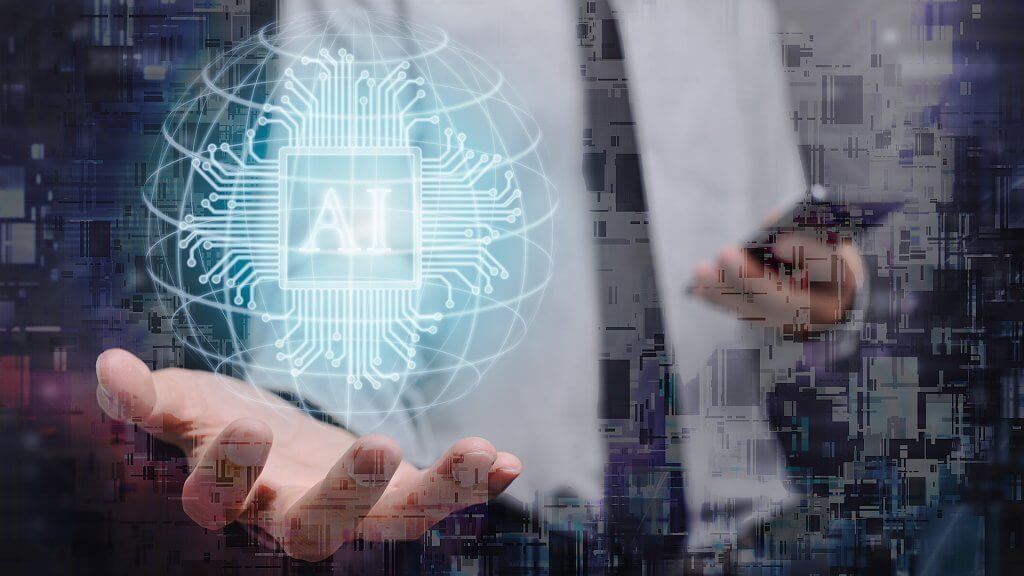
GPU and Cloud Cost Considerations
The rise of generative AI has put pressure on cloud computing expenses and GPU demand. As more organizations adopt AI, the need for cost optimization becomes paramount. Techniques like quantization, pruning, and model compression help reduce computational costs.
Think of it like managing your monthly expenses. Just as you might cut back on unnecessary subscriptions or find more affordable alternatives, businesses need to optimize their AI investments. By using techniques to make models more efficient, they can get the most bang for their buck.
Imagine you’re a startup founder looking to incorporate AI into your product. You’re excited about the possibilities, but you’re also worried about the costs. Training and deploying AI models can be expensive, especially if you need to use powerful GPUs in the cloud.
This is where techniques like quantization and pruning come in. Quantization involves reducing the precision of the numbers used in the model, which can significantly reduce the computational resources needed. It’s like simplifying a complex recipe by using fewer ingredients while still maintaining the flavor.
Pruning, on the other hand, involves removing unnecessary or redundant parts of the model. It’s like trimming the fat from a budget, getting rid of expenses that don’t contribute much to the bottom line. By pruning the model, you can make it smaller and faster without sacrificing too much accuracy.
But it’s not just about reducing costs. These techniques also have important implications for sustainability and accessibility. By making AI models more efficient, we can reduce their carbon footprint and make them more accessible to organizations with limited resources.
However, optimizing models for cost and efficiency is not a one-size-fits-all solution. It requires careful consideration of the specific use case, performance requirements, and available resources. Organizations need to strike the right balance between cost, speed, and accuracy.
Moreover, there are trade-offs to consider. Quantization and pruning can sometimes result in a slight decrease in model performance. It’s important to evaluate these trade-offs and make informed decisions based on the specific needs of the application.
Despite the challenges, the focus on cost optimization in AI is a positive trend. It encourages innovation and creative problem-solving, pushing the boundaries of what’s possible with limited resources. As more organizations adopt AI, finding ways to make it more efficient and affordable will be crucial for widespread adoption and impact.
So, the next time you’re considering incorporating AI into your business, don’t let the costs discourage you. With the right techniques and mindset, you can optimize your AI investments and get the most value for your money. And as we continue to push for more efficient and sustainable AI, let’s keep in mind the ultimate goal: making this transformative technology accessible and beneficial for all.
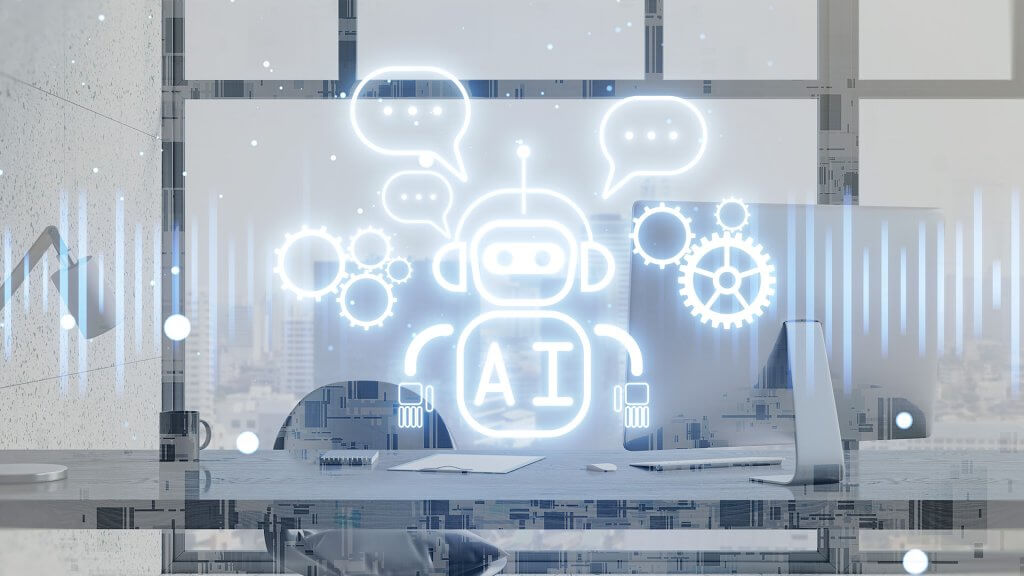
The Power of Custom Local Models
While cloud-based AI services offer convenience, there are advantages to training custom models locally. By using proprietary data and unique use cases, organizations can create AI solutions tailored to their specific needs. This approach also ensures greater control over sensitive data and compliance with regulations.
Imagine a healthcare provider that wants to develop an AI system for analyzing patient records. By training the model on their own data, they can ensure patient privacy and adhere to strict healthcare regulations. Open-source frameworks and pre-trained models make it easier than ever to build custom AI solutions.
Think about a retail company that wants to personalize product recommendations for its customers. With a custom local model, they can train the AI on their specific product catalog, customer preferences, and purchase history. This allows them to create a highly targeted and effective recommendation system that drives sales and improves customer satisfaction.
But the benefits of custom local models go beyond just performance. They also provide greater control and ownership over the AI system. When you train a model on your own data, you have complete visibility into how it works and what it’s learning. This transparency is crucial for building trust and accountability in AI.
Moreover, custom local models can help ensure compliance with data privacy regulations. In industries like healthcare and finance, there are strict rules around how sensitive data can be used and shared. By keeping the data and model training in-house, organizations can maintain control and meet these regulatory requirements.
However, building custom local models is not without its challenges. It requires a certain level of technical expertise and resources to train and deploy these models effectively. Organizations need to have the right infrastructure, data pipelines, and skilled personnel in place.
Additionally, there are trade-offs to consider. While custom local models provide greater control and specificity, they may not have access to the same vast amounts of data and computing power as cloud-based services. It’s important to weigh the benefits and limitations based on the specific use case and available resources.
Despite the challenges, the power of custom local models is undeniable. They offer a way for organizations to harness the full potential of AI while maintaining control, privacy, and compliance. As more industries look to adopt AI, the ability to build tailored solutions will become increasingly important.
So, whether you’re a healthcare provider looking to improve patient care or a retailer seeking to boost sales, consider the power of custom local models. With the right tools and approach, you can create an AI system that is perfectly suited to your unique needs and challenges. And as we continue to push the boundaries of what’s possible with AI, let’s remember the importance of keeping it local and under our control.
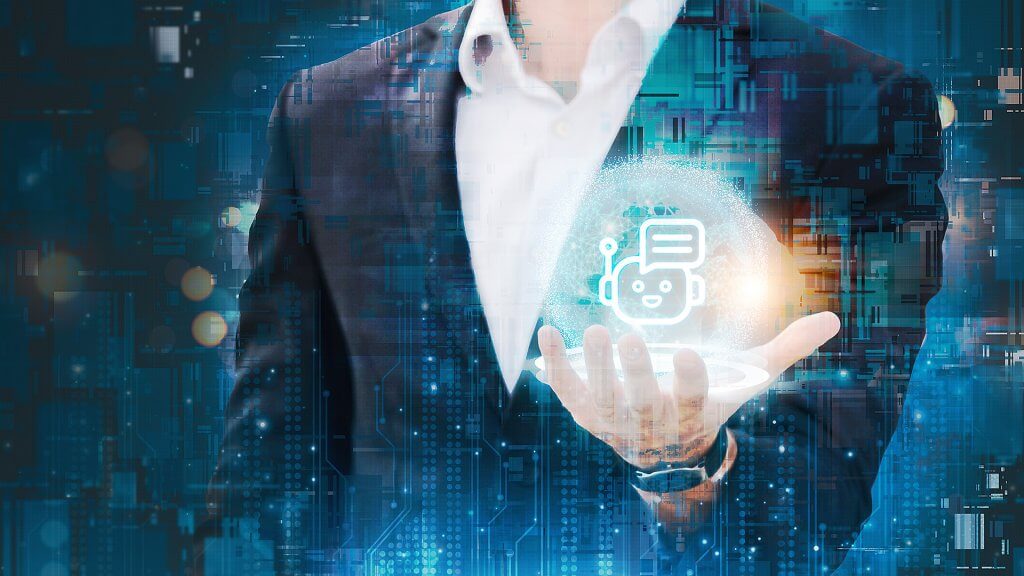
Virtual Agents and Task Automation
Conversational AI agents, or virtual agents, are becoming increasingly sophisticated. They can handle complex tasks, automate processes, and provide personalized assistance. The benefits for businesses are numerous, including increased productivity, reduced errors, and improved customer experiences.
Picture a virtual agent that can assist customers with booking appointments, answering questions, and even processing transactions. It’s like having a 24/7 customer support representative that never takes a break. By automating routine tasks, human employees can focus on more complex and creative work.
Imagine you’re a busy HR manager overwhelmed with employee inquiries about benefits, vacation policies, and payroll. With a virtual agent, you could automate many of these routine questions and tasks. Employees could interact with the virtual agent to get instant answers, freeing up your time to focus on more strategic initiatives.
But virtual agents aren’t just for customer support. They can also be used to automate internal processes and workflows. For example, a virtual agent could assist with onboarding new employees, guiding them through the necessary paperwork and training materials. It could even answer common questions and provide personalized recommendations based on the employee’s role and location.
In the healthcare industry, virtual agents can play a crucial role in patient care. They can help patients schedule appointments, provide medication reminders, and even monitor symptoms. By automating these tasks, healthcare providers can improve patient engagement and outcomes while reducing the burden on staff.
However, implementing virtual agents is not as simple as flipping a switch. It requires careful planning, design, and training to ensure the virtual agent can handle the complexity of real-world interactions. Organizations need to choose the right platform, define clear use cases, and continuously monitor and refine the virtual agent’s performance.
There are also important considerations around ethics and transparency. As virtual agents become more human-like, it’s crucial that users understand they are interacting with a machine. Organizations must be transparent about the capabilities and limitations of their virtual agents to avoid confusion or disappointment.
Despite the challenges, the potential of virtual agents and task automation is enormous. By leveraging the power of conversational AI, organizations can unlock new levels of efficiency, productivity, and customer satisfaction. As the technology continues to advance, we can expect to see virtual agents taking on even more sophisticated tasks and becoming an integral part of our daily lives.
So, the next time you interact with a virtual agent, whether it’s to book a flight or check your account balance, take a moment to appreciate the incredible technology behind it. And as we continue to push the boundaries of what’s possible with conversational AI, let’s remember to do so with empathy, transparency, and a focus on creating value for all.
The Impact of Increased AI Regulation
As AI becomes more pervasive, governments and regulatory bodies are taking notice. The European Union’s Artificial Intelligence Act is a notable example of emerging regulations. Striking a balance between fostering innovation and protecting citizens’ rights and intellectual property is crucial.
Businesses need to stay informed about the evolving regulatory landscape and embed responsible AI practices into their operations. Collaborating with regulators and other stakeholders can help shape the future of AI governance. It’s like being a responsible driver – by following the rules of the road, we can ensure a safer journey for everyone.
Imagine you’re a financial institution developing an AI system to assess loan applications. With increased regulation, you’ll need to ensure that your AI model is transparent, fair, and unbiased. This might involve conducting regular audits, documenting your data sources and algorithms, and providing clear explanations for how decisions are made.
But the impact of AI regulation goes beyond just compliance. It’s also about building trust with your customers and stakeholders. By embracing responsible AI practices, you can demonstrate your commitment to ethics and accountability. This can help differentiate your brand and build long-term loyalty.
However, navigating the regulatory landscape is not always straightforward. Different regions and industries may have varying requirements and standards. It’s important to stay proactive and engage with regulators to understand their expectations and provide input on how regulations can be effectively implemented.
Moreover, increased regulation can also create challenges for innovation. Striking the right balance between protecting rights and enabling progress is crucial. Overly restrictive regulations could stifle the development of beneficial AI applications, while lack of oversight could lead to unintended consequences.
That’s why collaboration between businesses, regulators, and other stakeholders is so important. By working together, we can create a framework for responsible AI that promotes innovation while safeguarding against risks. This might involve developing industry standards, sharing best practices, and fostering open dialogue.
As AI continues to evolve, so too will the regulatory landscape. Staying informed and adaptable will be key to thriving in this new environment. By embracing responsible AI practices and engaging proactively with regulators, businesses can not only mitigate risks but also unlock new opportunities for growth and impact.
So, as you navigate the impact of increased AI regulation, remember that it’s not just about ticking boxes. It’s about building a future where AI is used ethically, transparently, and for the benefit of all. By being a responsible steward of this transformative technology, you can help shape a world where AI and humanity thrive together.
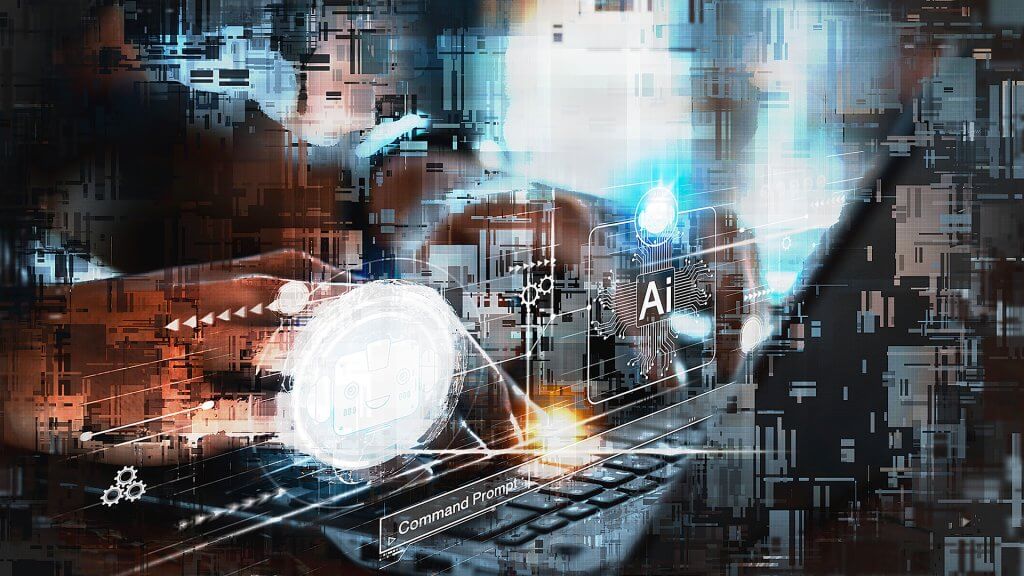
The Necessity of Responsible AI Use
The unauthorized or unethical use of AI within organizations, known as Shadow AI, poses significant risks. These include data breaches, copyright infringement, and reputational damage. Implementing comprehensive corporate governance and responsible AI policies is essential.
Imagine if an employee used AI to generate content that infringed on copyrights or contained biased language. The consequences could be severe, ranging from legal action to public backlash. By educating employees and fostering a culture of transparency and accountability, organizations can mitigate these risks.
Think about a marketing team that wants to use AI to analyze customer data and create targeted campaigns. Without proper governance and oversight, they might inadvertently use biased algorithms that discriminate against certain groups of customers. This not only violates principles of fairness and inclusivity but can also lead to significant reputational harm.
To prevent such scenarios, organizations must develop clear policies and guidelines around the use of AI. This includes defining what constitutes appropriate use, establishing processes for review and approval, and providing training and resources for employees. It’s like creating a code of conduct for AI – a shared understanding of what’s acceptable and what’s not.
But responsible AI use goes beyond just preventing misuse. It’s also about ensuring that AI is developed and deployed in a way that aligns with organizational values and societal expectations. This means considering the ethical implications of AI from the very beginning and embedding principles of fairness, transparency, and accountability into every stage of the AI lifecycle.
One way to do this is through the use of AI ethics frameworks and assessment tools. These provide a structured approach for evaluating the potential risks and benefits of AI systems and ensuring that they meet certain ethical standards. By using these tools, organizations can proactively identify and mitigate potential issues before they arise.
Another important aspect of responsible AI use is collaboration and communication. Engaging with stakeholders, including employees, customers, and external experts, can provide valuable insights and perspectives on the ethical considerations of AI. It’s like having a diverse team of advisors to help guide your AI journey.
Moreover, being transparent about your AI practices can help build trust and credibility with your stakeholders. This might involve communicating about your AI policies, sharing information about how your AI systems work, and being open about any challenges or limitations. By fostering a culture of transparency, you can demonstrate your commitment to responsible AI use.
However, implementing responsible AI practices is not always easy. It requires leadership buy-in, resources, and a willingness to make tough decisions. There may be trade-offs between efficiency and ethics, or between short-term gains and long-term sustainability. Navigating these challenges requires a clear vision, strong values, and a commitment to doing what’s right.
Despite the challenges, the necessity of responsible AI use cannot be overstated. As AI becomes more powerful and prevalent, the risks of misuse and unintended consequences only grow. By proactively addressing these risks and embedding responsible practices into your organization, you can not only protect your own interests but also contribute to a more positive and trustworthy AI ecosystem.
So, as you consider the role of AI in your organization, make responsibility a top priority. Educate your employees, develop clear policies and guidelines, and foster a culture of transparency and accountability. By doing so, you can harness the power of AI while mitigating the risks, and help create a future where AI is used for the benefit of all.
The AI trends for 2024 present both opportunities and challenges for businesses and society. From the rise of multimodal AI to the impact of increased regulation, navigating this landscape requires a proactive and responsible approach. By setting realistic expectations, optimizing costs, and prioritizing ethics, we can harness the power of AI for good.
As we shape the future of AI, it’s up to us to ensure that it benefits humanity as a whole. This means being mindful of the consequences of our actions and always striving to use AI in a way that promotes fairness, transparency, and accountability. Together, we can create an AI-powered future that is both innovative and inclusive.
The key takeaways from this article are:
1. Moving beyond the hype and setting realistic expectations for AI capabilities.
2. Embracing the potential of multimodal AI for more natural and intuitive interactions.
3. Striving for smaller, more efficient AI models that are accessible and sustainable.
4. Optimizing costs and resources through techniques like quantization and pruning.
5. Leveraging the power of custom local models for control, privacy, and compliance.
6. Harnessing virtual agents and task automation for increased productivity and customer satisfaction.
7. Navigating the impact of increased AI regulation through responsible practices and collaboration.
8. Prioritizing responsible AI use to mitigate risks and build trust with stakeholders.
As we look towards 2024 and beyond, it’s clear that AI will continue to transform industries, economies, and societies. The question is not whether AI will shape our future, but how we will shape the future of AI. Will we use it to create a world that is more equitable, sustainable, and fulfilling? Or will we let it exacerbate existing inequalities and challenges?
The choice is ours. By staying informed, engaged, and committed to responsible AI practices, we can create a future that we can all be proud of. A future where AI is not just a tool, but a partner in our journey towards a better world.
So let us embrace the opportunities and challenges of AI with courage, curiosity, and compassion. Let us use our human ingenuity to guide and shape this transformative technology, and let us never lose sight of the values and aspirations that make us human.
The future is ours to create, and with responsible AI as our ally, there’s no limit to what we can achieve.