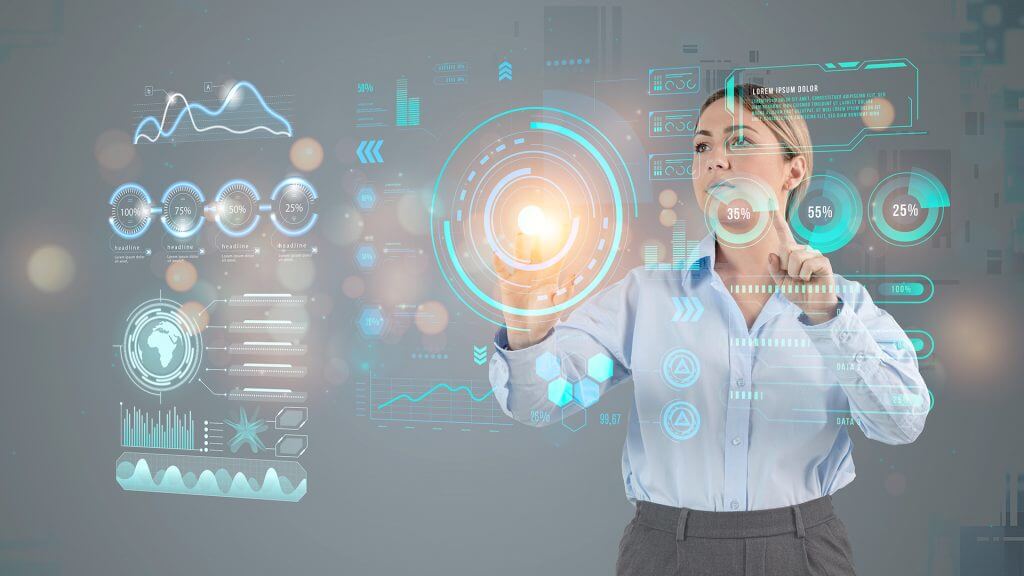
Advancements and Challenges in Artificial Intelligence
Artificial Intelligence (AI) is rapidly evolving, impacting various sectors from entertainment to politics. Understanding its current state and future trajectory is crucial for businesses and individuals alike.
The Vision and Future of AI
AI continues to reshape industries with innovative applications. In the entertainment sector, for instance, generative AI has revolutionized filmmaking, with major studios like Paramount and Disney employing AI for tasks like lip-syncing and special effects. AI is also extensively used in marketing and training, demonstrating its versatility across different fields.
The Quest for AGI
The pursuit of Artificial General Intelligence (AGI) is a key focus in the AI community. AGI represents a future where AI systems can understand, learn, and apply intelligence comparable to human cognition. This quest, while ambitious, faces numerous challenges, including the increasing demand for specialized hardware like GPUs. The shortage of GPUs, essential for running sophisticated AI algorithms, is a significant concern. Companies and countries are racing to secure these resources, underscoring the competitiveness in AI advancements. Efforts are underway to develop low-power alternatives to current GPUs, which could democratize access to AI technologies and foster more widespread innovation.
AI’s expansion into more practical applications is another area of growth. The transition from simple conversational interfaces to AI agents capable of performing tasks like making reservations or planning trips marks a significant leap in utility. This trend towards more helpful and interactive AI agents is expected to continue, with advancements in processing power opening the door to more complex applications, such as video analysis. Such developments could lead to AI systems with a deeper understanding of the world, as they process information captured in real-time, without human curation.
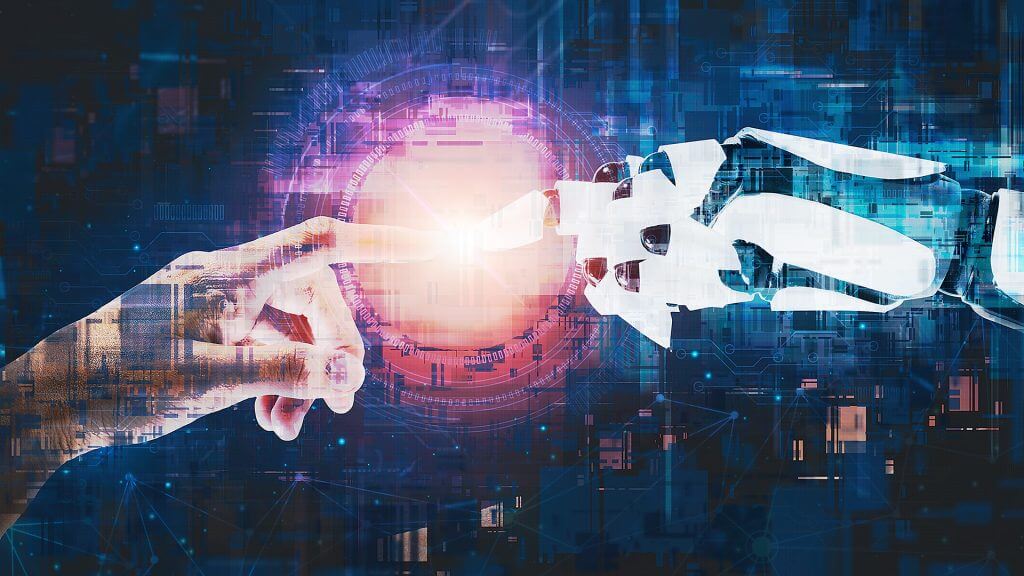
Contemporary Challenges in AI
AI’s rapid advancement raises ethical concerns, especially in the context of data privacy and misuse. For example, medical service providers handle vast amounts of sensitive data, and breaches in AI systems can have severe consequences, with personal data potentially being exposed on a large scale. This underlines the critical need for robust cybersecurity measures and innovative solutions to protect sensitive information.
Overcoming Bias and Data Scarcity
Bias in AI systems is a pressing issue. The quality of AI largely depends on the data it’s trained on, which is often limited and biased. This can skew AI’s understanding and representation of diverse populations. Furthermore, data scarcity, exacerbated by stringent regulations on data use, poses challenges for global AI applications. Developing algorithms that can efficiently address these biases and operate effectively with limited data is crucial for creating more equitable and functional AI systems.
While AI presents exciting opportunities for innovation and utility, it also poses significant challenges that need to be addressed to ensure its ethical and effective deployment. The future of AI lies in not just advancing its capabilities but also in responsibly navigating its societal impacts.
AI in Action: Real-World Applications and Limitations
AI’s journey from language-based models to multi-modal systems marks a significant advancement in its capabilities. These multi-modal models are not just limited to processing text but can analyze and generate images, sounds, and other forms of data. This evolution broadens AI’s applicability across various industries, from autonomous vehicles using image and LIDAR data to detect driver fatigue, to insurance companies analyzing claims through a mix of documents, images, and videos. However, this expansion also highlights the need for increased human involvement to ensure these systems remain relevant and attuned to real-world complexities.
AI’s Practical Capabilities and Constraints
AI today showcases remarkable abilities but also faces notable limitations. One such constraint is the challenge of generative AI in creating content that’s both original and compliant with copyright laws. High-profile lawsuits have been filed against major AI developers, including OpenAI and Microsoft, for alleged copyright infringement. This demonstrates the complex legal landscape AI must navigate as it continues to evolve.
Additionally, the use of AI in creating deepfakes for marketing and training, while innovative, raises ethical concerns. The ease of creating deepfakes can lead to misinformation and misuse in sensitive areas like elections, challenging the ability to discern reality online.
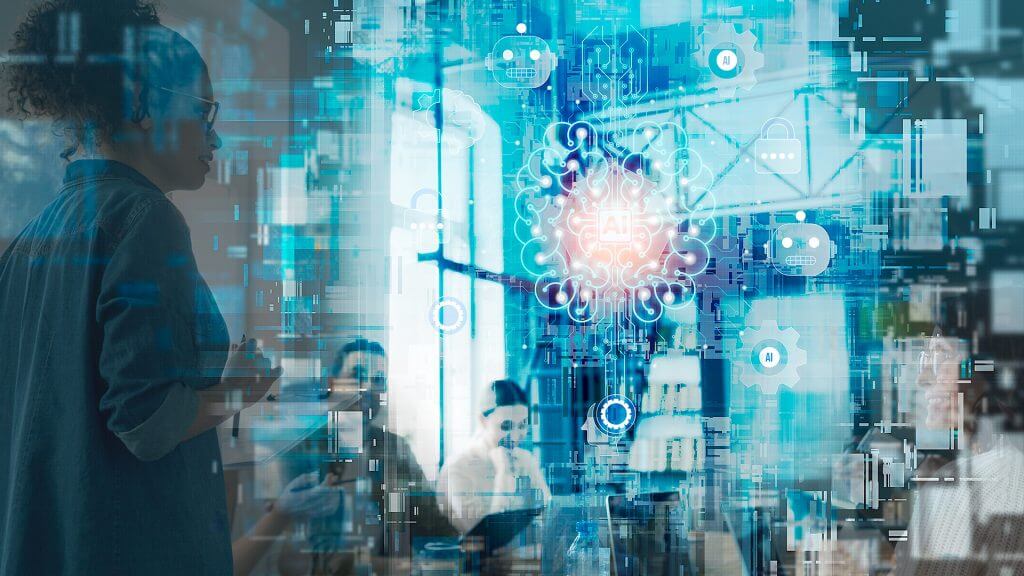
The Inner Workings of AI
AI’s approach to understanding complex human concepts is still a work in progress. The development of generalized AI systems, capable of performing a variety of tasks in unknown environments, is proceeding slowly. The “coffee test,” suggested by Steve Wozniak, exemplifies this challenge. It involves an AI system making a cup of coffee in an unknown household, requiring the AI to interpret unfamiliar settings and operate unknown equipment, a task far beyond the current capabilities of AI.
AI’s Mimicry of Human Reasoning
AI’s ability to mimic human thought processes is advancing but still faces significant hurdles. Current AI models, including large language models (LLMs), are being scrutinized for their limitations, such as difficulty with complex calculations or emergent behaviors. The trend is shifting towards a mixture of specialized, smaller models that may be more efficient and easier to update than monolithic models. However, there’s a growing realization that these systems often lack explainability, making it challenging to understand how AI reaches certain conclusions or decisions, especially in critical areas like finance or criminal justice.
While AI continues to advance and find new applications, it also confronts significant challenges. These include legal and ethical issues, limitations in understanding and adapting to complex real-world scenarios, and the ongoing struggle to create more generalized, transparent, and explainable AI systems.
Driving AI Forward: Data and Training Strategies
In AI development, the role of data and model scale is paramount in enhancing AI ‘intelligence’. As we head into 2024, synthetic data is predicted to become a primary resource for training AI models, with more than 60% of AI training data being synthetic by the end of the year. This shift towards synthetic data signifies a move from relying on real-world data to AI-generated data, which can fill gaps in areas like medical imaging or disease patterns. This change is part of a broader trend towards data-centric AI, where data management technologies aim to address challenges such as accessibility, volume, privacy, security, and complexity. In essence, the quality and scale of data significantly influence the capabilities and evolution of AI systems.
Training AI: From Basics to Advanced Skills
AI training involves various stages, from pre-training to post-training methodologies. Pre-training often includes the use of large-scale datasets to develop foundational capabilities, while post-training involves fine-tuning these models for specific applications. The growing importance of cloud-based infrastructure in this process is evident, as organizations continue to migrate to cloud-based data and analytics infrastructure. This shift, however, is accompanied by a more cost-conscious approach due to economic considerations. The trend indicates a movement towards more flexible and cost-effective lakehouse architectures over traditional data warehouses, aligning with decentralized and adaptable data management strategies.
Integrating AI Systems for Advanced Solutions
The integration of various AI models is crucial for developing comprehensive solutions. In 2024, we’re seeing a cautious but steady exploration of generative AI in enterprise applications, primarily enhancing customer service through advanced chatbots and serving as co-pilots in various industries. Large language models (LLMs) remain central to this, with major cloud vendors like OpenAI dominating the space. However, the development of these LLMs by individual enterprises is rare due to the expertise and resources required, keeping the power of LLMs centralized among key players. The integration of AI systems in enterprises thus hinges on combining these advanced models with organizational data and processes, often facilitated by cloud platforms like Databricks and Snowflake.
The Role of the AutoGen Framework
The AutoGen framework represents a significant advancement in AI, focusing on multi-agent orchestration. This approach is about enabling different AI systems to work together harmoniously. The idea is to allow teams to use their own data definitions while ensuring translations across the organization. This concept embraces the diversity of data operations within different domains of an organization, creating interoperability between various local definitions. Such a framework is crucial in addressing the challenges posed by inconsistent data definitions, which is a major hurdle in AI applications like natural language queries. By allowing diversity in operational and domain languages within an organization and facilitating connectivity between these diverse elements, the AutoGen framework paves the way for more effective and nuanced AI integrations.
As we advance in 2024, the trends in AI are towards greater reliance on synthetic data for training, a cautious but strategic exploration of generative AI in enterprises, and the integration of AI systems through frameworks like AutoGen. The focus is on building adaptable, cost-effective, and ethically aligned AI solutions that can cater to the diverse needs of modern organizations.
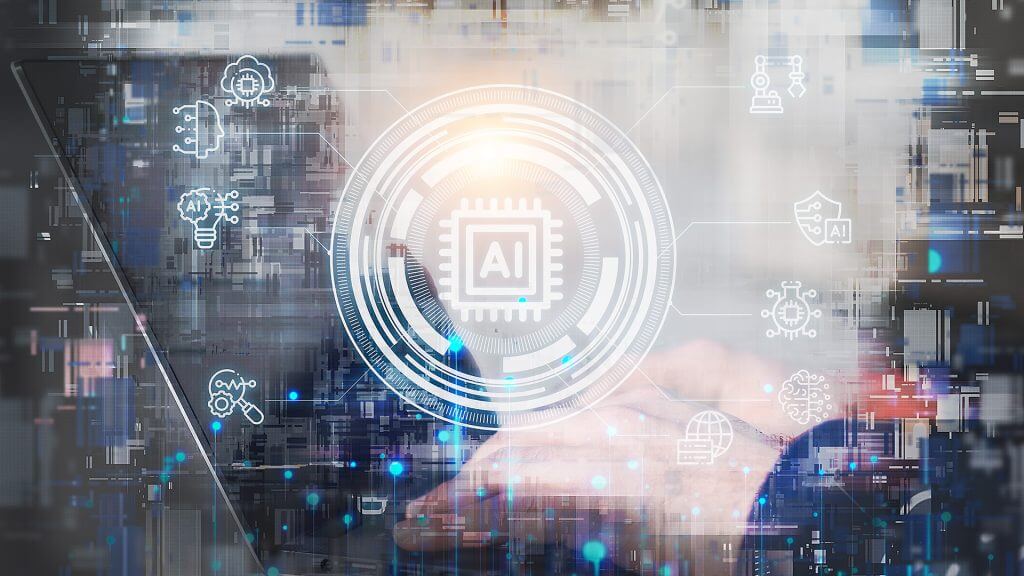
The Evolving Nature of AI Learning and Evaluation
Continuous learning is increasingly becoming a central aspect of AI development. This approach allows AI systems to constantly evolve and adapt, enhancing their capabilities over time. AI’s journey of continuous learning is characterized by the implementation of learning loops and feedback mechanisms, essential for the development of more sophisticated AI models. These mechanisms function similarly to human learning processes, where AI systems learn from their experiences and gradually improve their performance. This is evident in the work of AI researchers who emphasize the importance of continuous learning in AI systems, such as those at OpenAI, who focus on developing safe and effective AI technologies.
Redefining AI Evaluation and Progress
Evaluating and understanding AI development is shifting towards more dynamic and complex methodologies. Traditional metrics and evaluation techniques are being rethought to better align with the evolving capabilities of AI systems. This shift includes a focus on more nuanced and comprehensive evaluation methods that consider a wide range of factors, from performance metrics toethical considerations. As AI systems become more integrated into various sectors, the need for more robust and versatile evaluation frameworks becomes imperative. This includes assessing how AI models adapt and learn over time through feedback loops and continuous learning processes.
AI’s Societal Impact and Ethical Considerations
The focus is on building adaptable, cost-effective, and ethically aligned AI solutions that can cater to the diverse needs of modern organizations.As AI technologies become more advanced, their impact on various aspects of society, including economic, social, and ethical dimensions, grows. The development and deployment of AI systems are not isolated technical endeavors but are deeply embedded within societal contexts. This includes considering how societal norms, values, and challenges shape AI development and how AI, in turn, influences these aspects. Researchers and developers are increasingly aware of the need to consider these societal factors in AI design and implementation to ensure that AI technologies are beneficial and do not exacerbate existing societal issues.
Ethical Responsibilities in AI Advancement
The ethical dimension of AI development is a critical area of focus. As AI systems become more capable, the responsibility to ensure their ethical use and deployment becomes increasingly important. This includes addressing issues related to data privacy, bias, fairness, transparency, and accountability. The AI community is actively engaged in developing frameworks and guidelines to navigate these ethical challenges. These efforts are crucial in ensuring that AI technologies are developed and used in ways that align with human values and societal norms, avoiding unintended negative consequences. One example of this is the innovative HuGE AI breakthrough, which uses crowdsourced feedback in robot training, emphasizing the alignment of AI agents with human values.
The field of AI is experiencing significant developments in terms of learning mechanisms, evaluation methods, societal impact, and ethical considerations. Continuous learning and feedback loops are key to the evolution of AI, requiring dynamic evaluation strategies. Simultaneously, AI’s impact on society and the ethical responsibilities in its advancement remain pivotal areas of focus, ensuring AI develops in a manner that is beneficial and aligned with societal values.
The landscape of Artificial Intelligence (AI) is rapidly evolving, marked by significant advancements in learning mechanisms, ethical considerations, and societal impacts. Key insights from recent developments highlight the importance of continuous learning and feedback loops in AI systems, facilitating their adaptation and improvement over time. This approach, as seen in OpenAI’s research, underscores the dynamic nature of AI evolution, moving away from static models to more fluid and responsive systems.
Another crucial aspect of modern AI is the focus on ethical responsibilities and societal impacts. As AI becomes more integrated into various sectors, it’s clear that its development is not just a technological endeavor but a societal one. Ethical considerations such as fairness, transparency, and accountability are at the forefront, ensuring AI aligns with human values and societal norms. Innovative approaches like the HuGE AI breakthrough, which incorporates crowdsourced feedback in robot training, exemplify the efforts to align AI with human values.
These discussions about AI’s continuous learning, ethical considerations, and societal impacts are not just academic; they are essential for guiding AI’s path forward. As AI technologies become more advanced and pervasive, the importance of understanding and addressing these aspects cannot be overstated.
The future outlook of AI is one that is intertwined with the fabric of society, necessitating a thoughtful and responsible approach to its development and implementation. By acknowledging and addressing these key areas, we can ensure that AI develops in a way that benefits society as a whole, steering clear of potential pitfalls and harnessing its full potential for positive impact.
As we continue to advance in the field of AI, it is crucial to maintain an open dialogue about these critical issues, ensuring that AI evolves not only in technical sophistication but also in ethical and societal harmony. Revolutionize your operations with AI. Get in touch.