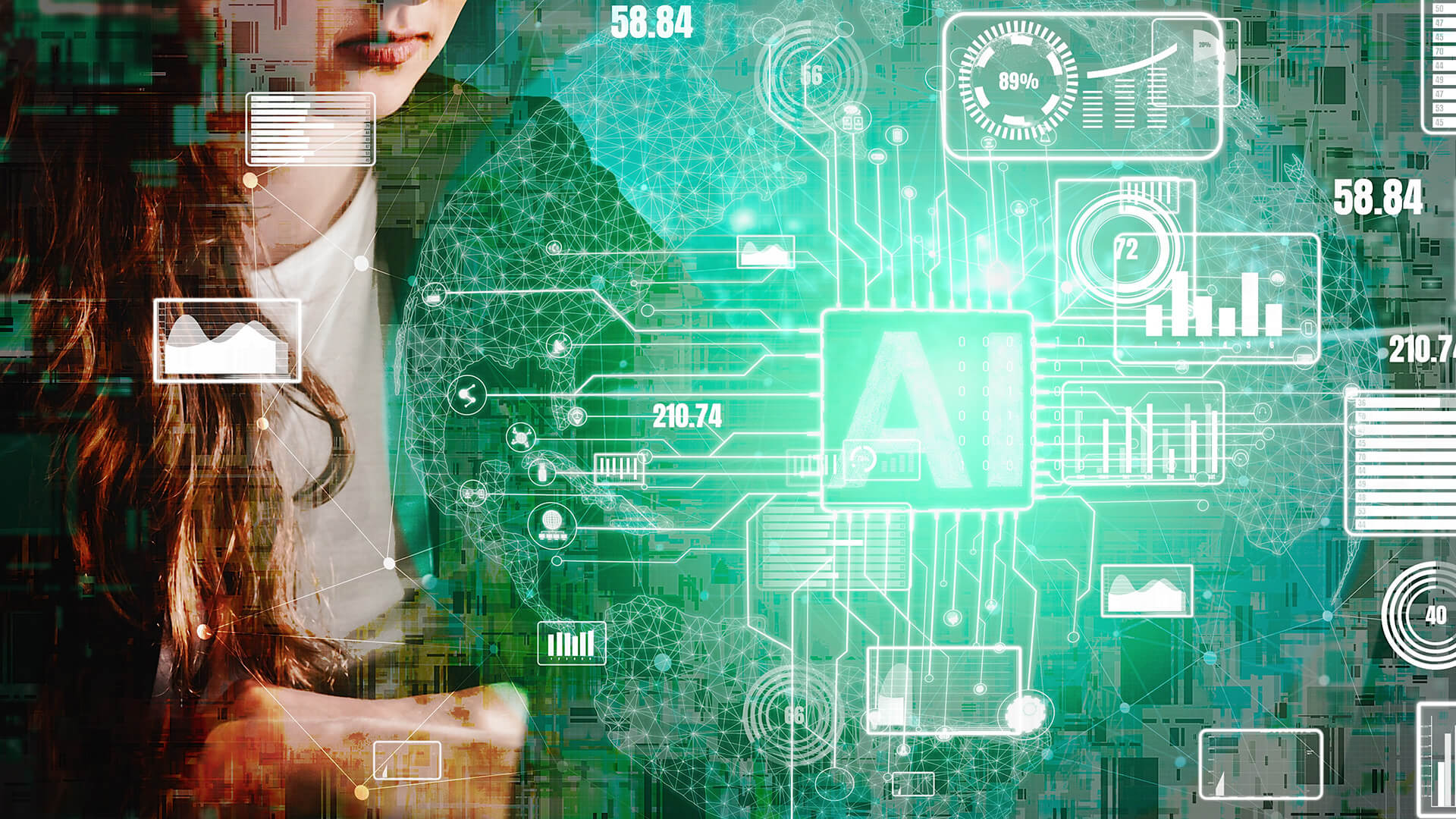
Envision a world where the overwhelming challenge of navigating through an endless sea of digital documents is a relic of the past. In our increasingly data-centric society, this formidable task is a daily reality for many professionals. Enter Azure AI Document Intelligence: a beacon of innovation in the realm of document processing. This breakthrough technology is not just an improvement but a complete overhaul of how we handle Retrieval Augmented Generation (RAG) and search processes.
This blog delves deep into the heart of Azure AI’s capabilities, unraveling its profound impact on document processing, and illuminating its wide array of practical applications. It’s a journey into a future where AI not only assists but transforms our interaction with information.
Exploring Azure AI Document Intelligence
Key Features and Functionalities
At the cutting edge of AI-driven document processing, Azure AI Document Intelligence emerges as a game-changer. This tool is not merely processing documents; it’s redefining the process. With its advanced algorithms, it delves into the depths of documents, extracting and categorizing critical data with an accuracy and efficiency that traditional methods can’t match.
The secret lies in its fusion of natural language processing and machine learning – a combination that enables it to understand the subtleties and nuances of human language, turning unstructured data into a wellspring of actionable insights.
Application Scenarios in Document Processing
The versatility of Azure AI Document Intelligence is its most striking feature, making it a valuable asset across a spectrum of industries. Legal firms, for instance, are transformed into bastions of efficiency as this tool swiftly sifts through case files and legal precedents, uncovering crucial information in a fraction of the time it would take manually. The healthcare sector, too, reaps enormous benefits.
Here, Azure AI becomes a vital tool in managing patient records and research data, not just streamlining administrative burdens but also opening new avenues in patient care and medical research. The implications are vast – from reducing operational costs to enhancing the quality of service and decision-making. Azure AI Document Intelligence is not just a tool; it’s a revolution in how industries interact with information.
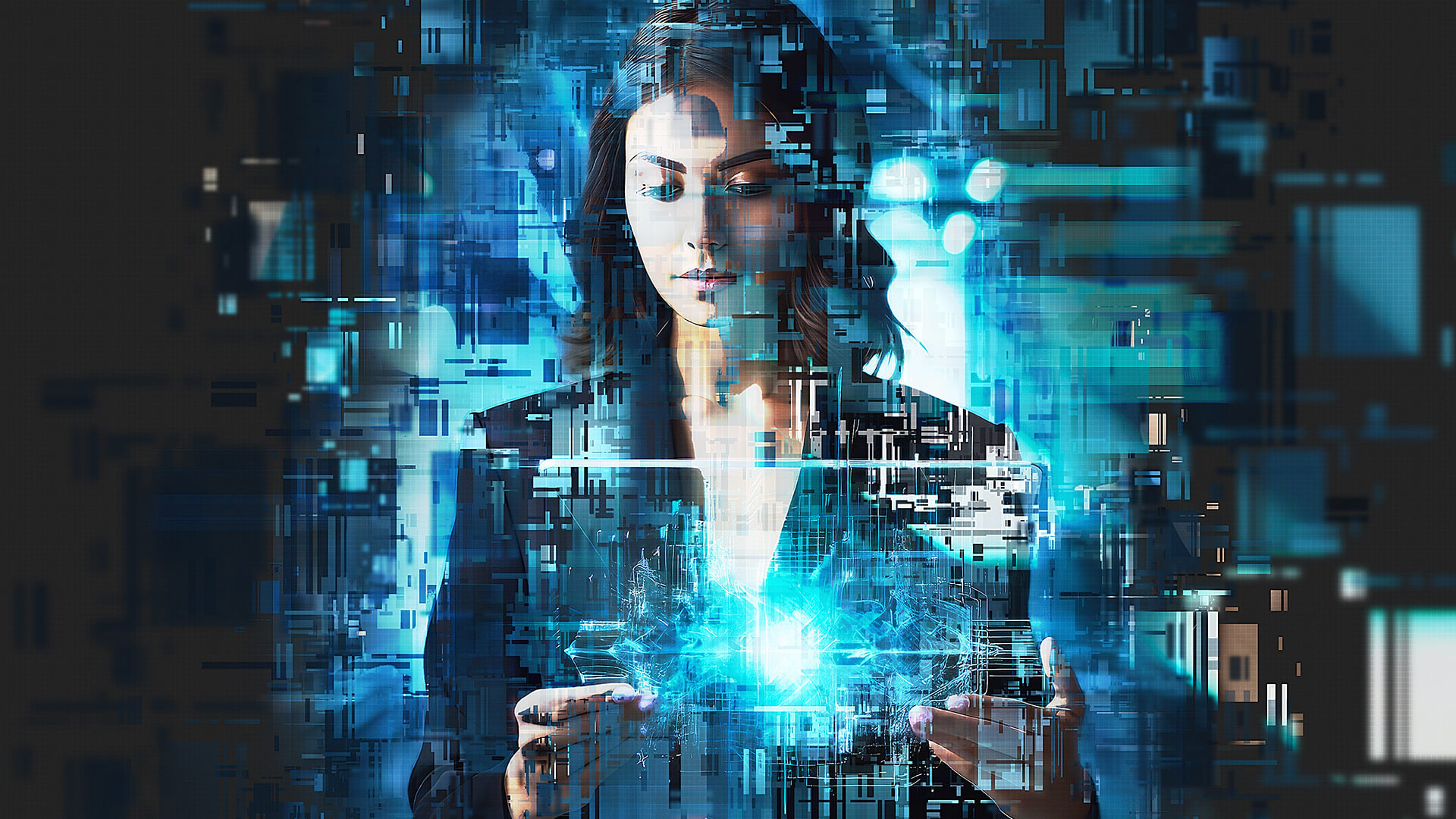
Understanding Retrieval Augmented Generation (RAG)
The advent of Retrieval Augmented Generation (RAG) marks a significant leap in the field of artificial intelligence, heralding a new era of information processing and retrieval. This innovative concept isn’t just an addition to the AI toolkit; it’s a fundamental reimagining of how AI interacts with and utilizes data.
The Concept of RAG in AI
At its core, RAG is a fusion of two distinct but complementary AI disciplines: information retrieval and generative AI models. This synergy allows AI systems to transcend traditional boundaries, enabling them to source and integrate external information dynamically. RAG is akin to giving AI the ability to research: it can reach into vast databases or document sets, extract relevant information, and use this to inform and enhance its responses. This isn’t just about finding data; it’s about understanding and utilizing that data in real-time to create more informed, accurate, and contextually rich outputs.
How RAG Enhances AI Search and Responses
The implications of RAG for AI search and response systems are profound. In customer service, for example, RAG transforms chatbots from simple query-responding machines into sophisticated virtual assistants capable of accessing and interpreting a company’s entire knowledge base. This means that when a customer asks a question, the chatbot isn’t limited to pre-programmed responses; it can provide answers that are not only precise but also deeply tailored to the context of the query.
This results in a dramatically improved customer experience – interactions become more meaningful, solutions more relevant, and customer satisfaction soars. RAG’s potential extends beyond customer service, infiltrating every domain where AI interacts with data, promising a future where AI’s ability to understand and respond to our needs is limited only by the breadth of available information.
Semantic Chunking and Its Importance
The evolution of document processing has reached a pivotal juncture with the advent of semantic chunking, a technique that stands in stark contrast to traditional methods. This innovative approach addresses the fundamental challenges inherent in conventional document chunking, paving the way for more efficient and meaningful data processing.
Challenges of Traditional Document Chunking
Conventional document chunking has often been a game of hit and miss, where the risk of fragmenting information and losing context is high. Traditional methods typically segment documents based on arbitrary criteria such as word count or page number, disregarding the natural flow and meaning of the content. This can lead to disjointed pieces of information that are out of context, making searches inefficient and data analysis incomplete.
The result? A cumbersome process that often requires additional human intervention to piece together fragmented information, leading to increased time, effort, and the potential for error.
Advantages of Semantic Chunking in Azure AI
In contrast, semantic chunking in Azure AI represents a paradigm shift. This advanced method organizes data based on its inherent meaning, not just superficial criteria. By understanding the context and nuances of language, Azure AI can intelligently segment documents into coherent chunks that maintain the integrity of the information.
This logical grouping ensures that when data is retrieved or analyzed, it is done so in a way that preserves and respects the original context. The benefits are manifold: searches become more targeted and efficient, data analysis gains depth and accuracy, and overall, the system aligns more closely with human understanding and logic.
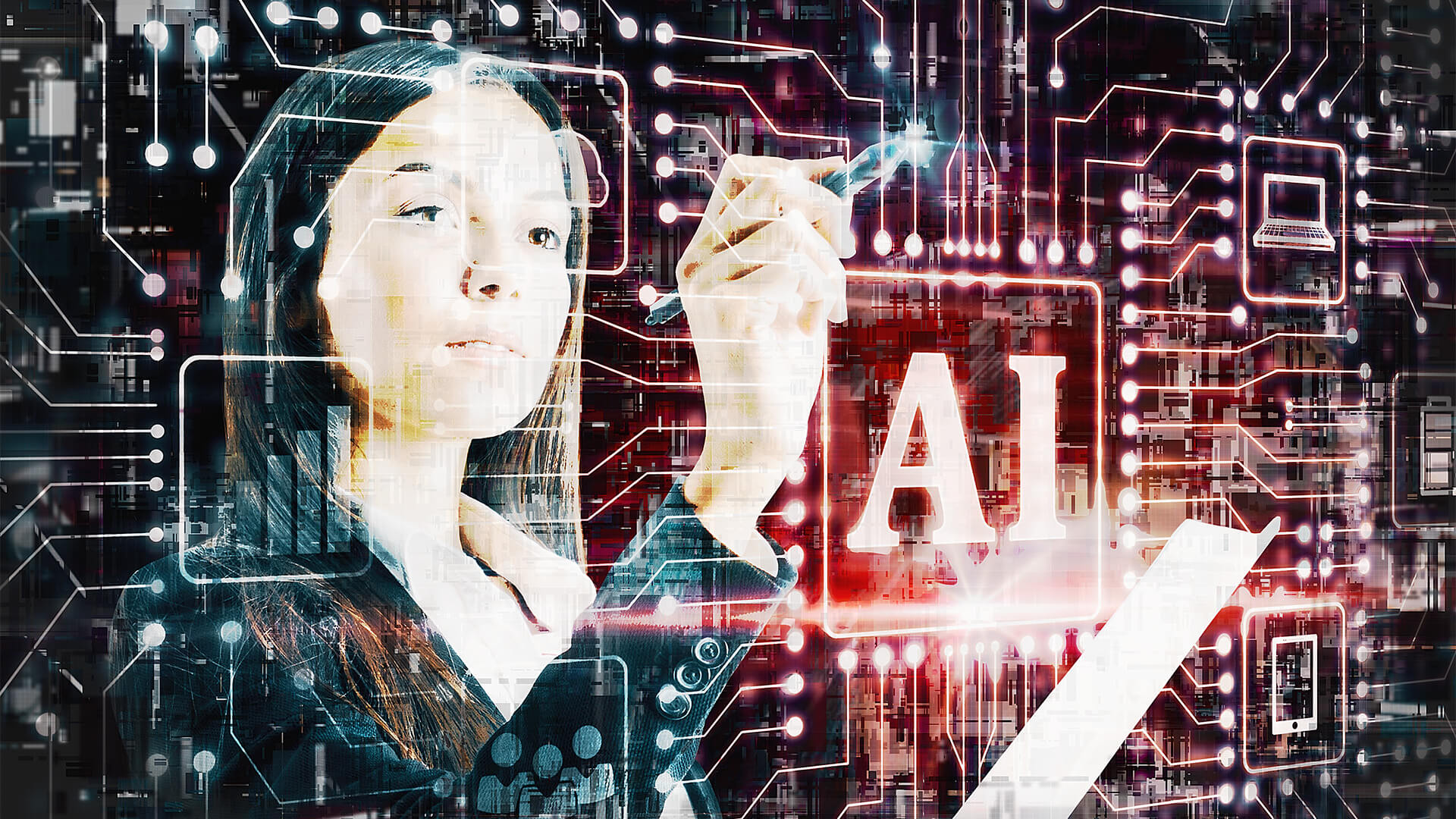
Practical Implementation of Azure AI and RAG
Embarking on the journey to implement Azure AI and RAG in your business can be transformative, but it requires careful planning and execution. The following guide offers a roadmap to effectively integrate these cutting-edge technologies into your existing systems.
Step-by-Step Guide to Implementation
- Define the Scope: Begin by clearly defining what you want to achieve with Azure AI and RAG. Identify the specific areas or processes within your business that will benefit from enhanced document processing and search capabilities.
- Data Preparation: Prepare your data for processing. This involves organizing your documents and ensuring they are in a format that is compatible with Azure AI.
- Customize and Train the Models: Customize the Azure AI and RAG models to suit your specific business needs. This may involve training the models on your specific data sets to ensure they accurately understand and process your documents.
- Integration: Integrate these tools with your existing systems. This step is crucial and may require technical expertise to ensure seamless integration without disrupting current operations.
- Testing and Evaluation: Rigorously test the system to ensure it meets your requirements. Evaluate its performance and make adjustments as necessary.
- Deployment and Training: Once testing is complete, deploy the system across your organization. Train your staff on how to use the new tools effectively.
- Continuous Improvement: Continuously monitor and update the system to improve its accuracy and efficiency over time.
Case Studies and Success Stories
The practical benefits of Azure AI and RAG are not hypothetical; they are evidenced in numerous success stories. An e-commerce giant, for instance, implemented these tools to revamp its product search functionality. The result was a dramatic improvement in search accuracy and efficiency, leading to increased customer satisfaction and a noticeable uptick in sales.
Such real-world applications underscore the transformative potential of these technologies, offering a glimpse into a future where AI-driven solutions not only streamline operations but also drive business growth and customer engagement.
Practical Applications/Real-World Implications
The integration of Azure AI Document Intelligence with Retrieval Augmented Generation (RAG) is not just a technological advancement; it’s a catalyst for a paradigm shift in information management and retrieval. This powerful combination is set to redefine efficiency and accuracy in a multitude of real-world scenarios.
In the realm of digital libraries, for example, this integration transforms the way users interact with vast repositories of knowledge. Azure AI and RAG enable a search functionality that is not just about finding information but about understanding the intent behind each query. This means users can navigate through extensive digital collections to pinpoint precise information swiftly and accurately, a significant boon for researchers, academics, and students alike.
In the corporate world, the impact is equally transformative. Enterprises inundated with documents can leverage this technology to streamline their document management processes. Azure AI’s ability to intelligently categorize and process information, combined with RAG’s enhanced search capabilities, means that valuable data can be retrieved and utilized more efficiently than ever before. This leads to a substantial reduction in the time and resources traditionally required for document management, thereby enhancing productivity and decision-making processes across various business functions.
Critical Analysis or Discussion
While Azure AI Document Intelligence stands as a formidable tool in the AI landscape, a critical analysis reveals areas where it can be juxtaposed with other document processing tools. Each tool comes with its unique strengths and limitations, and the choice often depends on specific use cases and requirements. Future enhancements in Azure AI, particularly in areas like natural language understanding and context-aware processing, could further elevate its capabilities, potentially setting new benchmarks in document processing and AI-enhanced search.
Azure AI Document Intelligence, in conjunction with RAG, is playing a pivotal role in revolutionizing the search and document processing landscape. As we peer into the future, it’s intriguing to ponder how continued advancements in AI will further reshape the fields of document processing and knowledge management. The possibilities are as vast as they are exciting, opening new horizons for efficiency and innovation.
For those who find themselves at the crossroads of curiosity and innovation, seeking to delve deeper into the capabilities and applications of Azure AI Document Intelligence, AI50 stands as a beacon of guidance and expertise. Reaching out to AI50 can provide not only in-depth insights but also tailored solutions that harness the full potential of Azure AI to meet specific business needs and challenges.
Frequently Asked Questions
Azure AI Document Intelligence is an AI-driven tool that enhances document processing and search capabilities using advanced algorithms and machine learning techniques.
RAG combines information retrieval with AI’s generative capabilities, allowing AI systems to pull relevant data from databases or documents, thereby improving the context and accuracy of responses.
Semantic chunking organizes data based on meaning, improving the efficiency and effectiveness of data retrieval and analysis.
These technologies can significantly improve document processing efficiency, enhance search capabilities, and provide more accurate, contextually relevant responses in various applications.